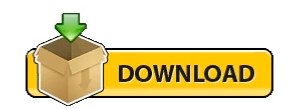
13.3.1 Core model operations: calculation and simulation. 13.2.4 Getting distribution bounds for a node. 13.2.3 Getting distribution parameter values for a node. 13.2.2 Getting information about a distribution. 13.2.1 Getting distributional information about a node. 13.2 Accessing information about nodes and variables. 13.1.3 Determining dependencies in a model. 13.1.1 Determining the nodes in a model.
13.1 The variables and nodes in a NIMBLE model. 12.2.1 Using registerDistributions for alternative parameterizations and providing other information. 12 Creating user-defined BUGS distributions and functions. 11.7 Calling uncompiled R functions from compiled nimbleFunctions. 11.5 Compiled nimbleFunctions pass arguments by reference. 11.4 Declaring argument and return types. 11.3 How NIMBLE handles types of variables. 11.2.9 ‘nim’ synonyms for some functions. 11.2.8 Optimization: optim and nimOptim.
11.2.7 Checking for user interrupts: checkInterrupt.11.2.5 Flow control: if-then-else, for, while, and stop.11.2.1 Finding help for NIMBLE’s versions of R functions.11.2 R functions (or variants) implemented in NIMBLE.11.1 Introduction to simple nimbleFunctions.10.1 Bayesian nonparametric mixture models.9.2 Proper Gaussian CAR model: dcar_proper.9.1 Intrinsic Gaussian CAR model: dcar_normal.8.2.1 Estimating the asymptotic covariance From MCEM.8.2 Monte Carlo Expectation Maximization (MCEM).8.1 Particle filters / sequential Monte Carlo and iterated filtering.8 Sequential Monte Carlo, Particle MCMC, Iterated Filtering, and MCEM.7.12 Comparing different MCMCs with MCMCsuite and compareMCMCs.7.10.2 Customized log-likelihood evaluations: RW_llFunction sampler.7.9 Variable selection using Reversible Jump MCMC.7.5.2 Assessing the adaption process of RW and RW_block samplers.7.5.1 Measuring sampler computation times: getTimes.
7.4 User-friendly execution of MCMC algorithms: runMCMC. 7.2.2 Customizing the MCMC configuration. 7.1 One-line invocation of MCMC: nimbleMCMC. 6.2.2 Determining the nodes and variables in a model. 6.2 NIMBLE models are objects you can query and manipulate. 6.1.3 Making multiple instances from the same model definition. 6.1.2 Creating a model from standard BUGS and JAGS input files. 6.1.1 Using nimbleModel to create a model. 5.2.7 Truncation, censoring, and constraints. 5.2.5 Available BUGS language functions. 5.2.3 Vectorized versus scalar declarations. 5.2.1 Declaring stochastic and deterministic nodes. 5.1.3 Not-yet-supported features of BUGS and JAGS. 5.1.2 NIMBLE’s Extensions to BUGS and JAGS. 5.1.1 Supported features of BUGS and JAGS. 5.1 Comparison to BUGS dialects supported by WinBUGS, OpenBUGS and JAGS. 5 Writing models in NIMBLE’s dialect of BUGS. 4.5.4 Customizing compilation of the NIMBLE-generated C++. 4.4 Troubleshooting installation problems. 4.2 Installing a C++ compiler for NIMBLE to use. 3.2 nimbleFunctions for writing algorithms. 3.1 NIMBLE adopts and extends the BUGS language for specifying models. 2.5 Creating, compiling and running a basic MCMC configuration.